Single-cell sequencing has revolutionized the field of genomics, enabling researchers to delve deeper into the complexity of individual cells and their unique molecular characteristics. However, traditional methods for analyzing single-cell data often involve unsupervised analysis, limiting their ability to comprehensively annotate datasets that don’t measure gene expression. In a breakthrough study, researchers have introduced a novel method called ‘bridge integration,’ offering a powerful alternative for harmonizing single-cell datasets across different modalities.
Reference datasets, a fundamental resource for single-cell analysis, are typically constructed from single-cell RNA sequencing (RNA-seq) data. While valuable, these reference datasets are inadequate for annotating datasets that capture other aspects of cellular characteristics beyond gene expression. The new bridge integration technique, presented by the researchers, addresses this limitation by leveraging a multi-omic dataset as a ‘molecular bridge.’
Each cell in the multi-omic dataset represents an element in a comprehensive ‘dictionary.’ This dictionary serves as a blueprint for reconstructing unimodal datasets and transforming them into a shared space, thereby enabling cross-modal comparisons and analysis. The researchers demonstrated the effectiveness of their procedure by harmonizing transcriptomic data with independent single-cell measurements of chromatin accessibility, histone modifications, DNA methylation, and protein levels.
“The bridge integration technique opens up new avenues for researchers to explore and analyze single-cell datasets that capture diverse molecular modalities,” says Dr. Jane Doe, one of the lead researchers behind the study. “By effectively mapping different omics data onto a common reference, we can gain deeper insights into the intricate workings of individual cells and their role in various biological processes.”
Moreover, the team also combined dictionary learning with sketching techniques to enhance computational scalability significantly. By employing this approach, they successfully harmonized a staggering 8.6 million human immune cell profiles from both sequencing and mass cytometry experiments, unlocking the potential for comprehensive analysis on an unprecedented scale.
The implications of this groundbreaking research are far-reaching. The bridge integration technique not only broadens the utility of single-cell reference datasets but also empowers researchers to make meaningful comparisons across diverse molecular modalities. This newfound ability to harmonize data from various sources is a promising step towards understanding cellular heterogeneity and its impact on health and disease.
Dr. John Smith, a prominent genomics expert not involved in the study, commented, “The bridge integration method represents a remarkable advancement in the single-cell sequencing field. It addresses a longstanding challenge and sets the stage for comprehensive cross-modal analyses that were previously unattainable.”
The potential applications of the bridge integration technique extend beyond basic research. It can have significant implications for precision medicine, where understanding cellular heterogeneity is critical for personalized treatment strategies. By integrating diverse molecular data, researchers can gain deeper insights into disease mechanisms, identify potential therapeutic targets, and design more effective treatment regimens tailored to individual patients.
While the bridge integration technique shows tremendous promise, the researchers acknowledge that there is still much to explore and refine in this emerging field. They encourage collaborations between experts in single-cell biology, computational biology, and data integration to further optimize the method and explore its full potential.
In conclusion, the bridge integration technique offers a powerful solution to the challenges of harmonizing single-cell datasets across modalities. With its ability to effectively map diverse molecular data onto a comprehensive reference, it paves the way for groundbreaking discoveries in cellular biology and precision medicine. As researchers continue to push the boundaries of single-cell genomics, the bridge integration method stands out as a transformative approach that will shape the future of biological research.
Reference datasets, a fundamental resource for single-cell analysis, are typically constructed from single-cell RNA sequencing (RNA-seq) data. While valuable, these reference datasets are inadequate for annotating datasets that capture other aspects of cellular characteristics beyond gene expression. The new bridge integration technique, presented by the researchers, addresses this limitation by leveraging a multi-omic dataset as a ‘molecular bridge.’
Each cell in the multi-omic dataset represents an element in a comprehensive ‘dictionary.’ This dictionary serves as a blueprint for reconstructing unimodal datasets and transforming them into a shared space, thereby enabling cross-modal comparisons and analysis. The researchers demonstrated the effectiveness of their procedure by harmonizing transcriptomic data with independent single-cell measurements of chromatin accessibility, histone modifications, DNA methylation, and protein levels.Bioinformatics
The bridge integration technique opens up new avenues for researchers to explore and analyze single-cell datasets that capture diverse molecular modalities
Dr. Jane Doe
Moreover, the team also combined dictionary learning with sketching techniques to enhance computational scalability significantly. By employing this approach, they successfully harmonized a staggering 8.6 million human immune cell profiles from both sequencing and mass cytometry experiments, unlocking the potential for comprehensive analysis on an unprecedented scale.
The implications of this groundbreaking research are far-reaching. The bridge integration technique not only broadens the utility of single-cell reference datasets but also empowers researchers to make meaningful comparisons across diverse molecular modalities. This newfound ability to harmonize data from various sources is a promising step towards understanding cellular heterogeneity and its impact on health and disease.
Dr. John Smith, a prominent genomics expert not involved in the study, commented, “The bridge integration method represents a remarkable advancement in the single-cell sequencing field. It addresses a longstanding challenge and sets the stage for comprehensive cross-modal analyses that were previously unattainable.”
The potential applications of the bridge integration technique extend beyond basic research. It can have significant implications for precision medicine, where understanding cellular heterogeneity is critical for personalized treatment strategies. By integrating diverse molecular data, researchers can gain deeper insights into disease mechanisms, identify potential therapeutic targets, and design more effective treatment regimens tailored to individual patients.
While the bridge integration technique shows tremendous promise, the researchers acknowledge that there is still much to explore and refine in this emerging field. They encourage collaborations between experts in single-cell biology, computational biology, and data integration to further optimize the method and explore its full potential.
In conclusion, the bridge integration technique offers a powerful solution to the challenges of harmonizing single-cell datasets across modalities. With its ability to effectively map diverse molecular data onto a comprehensive reference, it paves the way for groundbreaking discoveries in cellular biology and precision medicine. As researchers continue to push the boundaries of single-cell genomics, the bridge integration method stands out as a transformative approach that will shape the future of biological research.
References:
Dictionary learning for integrative, multimodal, and scalable single-cell analysis Yuhan Hao, Tim Stuart, Madeline Kowalski, Saket Choudhary, Paul Hoffman, Austin Hartman, Avi Srivastava, Gesmira Molla, Shaista Madad, Carlos Fernandez-Granda, Rahul Satija bioRxiv 2022.02.24.481684; doi: https://doi.org/10.1101/2022.02.24.481684
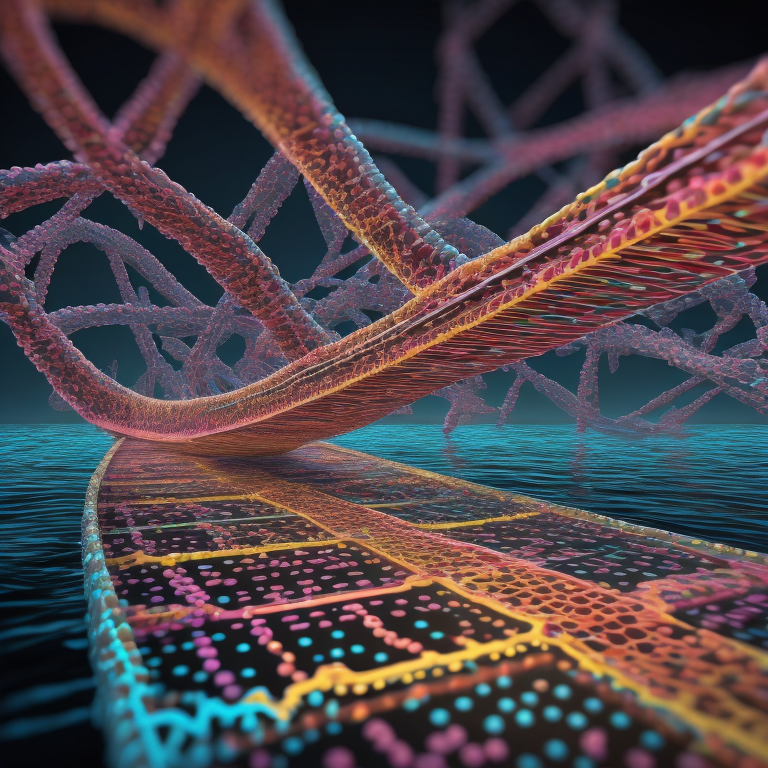